How AI is Driving the Returns Revolution
By Mike Ganz, Optoro
Reverse logistics software is one of the most powerful tools a retailer can invest in to cut costs, drive efficiency, and improve processing – especially with the peak holiday season ramping up. These returns platforms are driven by data science and automation technology to effectively receive and route returns, all while providing crucial data to retailers about their supply chains. Today, the best returns platforms operate using AI from end-to-end. So, let’s dive into how it works, best practices for leveraging this technology, and what’s on the horizon for AI.
WHAT IS AI?
Artificial Intelligence – or AI – is a broad term to describe any piece of technology that aims to simulate behavior typically exhibited by humans. The complexity of this technology’s applications range considerably, from something as straightforward as curating a personalized social media feed, to something as intricate as the computer vision technology that powers self-driving automobiles.
What unites these examples, however, is a common intent: creating efficiency in previously inefficient tasks. Although reverse logistics is still in its early days of AI innovation, we’re already seeing a wide array of applications all geared towards one central question: “How can we remove friction from the returns process?”
LEVERAGING AI METRICS TO IMPROVE REVERSE LOGISTICS
One way retailers are going about this goal is by onboarding AI solutions to assist them in making smarter routing decisions. Oftentimes in supply chains, inventory takes circuitous routes to its final destination, either because the decision maker doesn’t have full visibility into their network or isn’t considering all of that information at the time the decision is made. Graph theory – a branch of mathematics dedicated to identifying patterns in the relationships between different connected entities – is perfectly suited to solve this type of problem. Taking this approach, a retailer could map out the sequence of steps a return could go through (ex. shopper → warehouse → store vs. shopper → warehouse → original vendor), identify the costs associated with these sequences, and then apply any number of easy-to-implement algorithms to find the lowest cost route for a given return.
At scale across hundreds of millions of returns, this type of technology can generate insights (and cost savings) less advanced systems would not be able to. For example, many retailers – especially those selling goods at cheaper price points – end up taking a loss on a sizable percentage of their returns because their cost to process outweighs their resale or restock value. Graph algorithms can help identify more efficient options for these types of returns, such as customer keep – a returns option wherein eligible products and customers are offered a refund without needing to actually return their item. This option can help retailers cut transportation costs up to 15% by avoiding these unnecessary touches.1 That’s on top of the immense environmental benefit customer keep can generate, reducing CO2 emissions by 110,000 lbs for every 100,000 items returned.2
Aside from informing routing, leading retailers use AI to better understand the quality of the returns coming back into their warehouses. Products being returned still in their original packaging or appearing brand new can sell for up to 10x more on primary and secondary markets3 than ones coming back dirty or broken. However, historically there has not been a reliable system for efficiently determining an item’s condition. Natural Language Processing (NLP) models that can sort through customer comments and computer vision algorithms that aim to “inspect” items for scratches, dents, or tears, offer an efficient and accurate way to assess that crucial information.
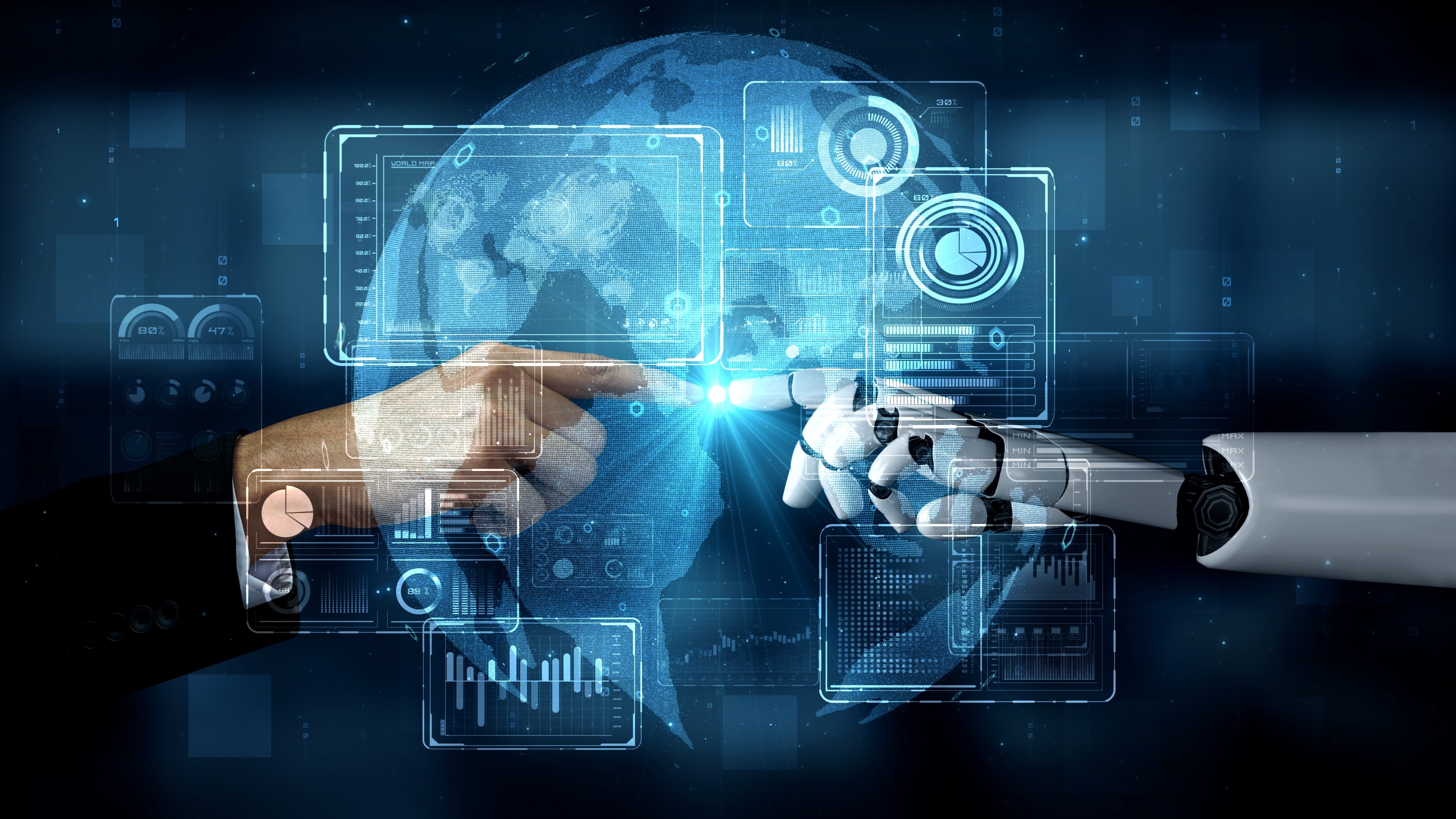
THE FUTURE OF AI
Advancement in computer vision – or a machine’s ability to simulate observing an object’s appearance and make decisions based on it – will bring substantial gains to the reverse logistics industry. We’re already seeing the impact this technology can have, from inspecting the quality of returned goods and assigning them conditions without human intervention, to the autonomous robotic systems that can navigate a warehouse floor, picking and placing items with surgical precision.
Recent progress in the field of reinforcement learning has also allowed the logistics world to better navigate the complex world of inventory fulfillment. Reinforcement learning stands out from other types of machine learning in that it is less about teaching a system to predict some outcome. Rather, it is about teaching that system how to be smart with choosing the decision it makes. One can see how this extends to fulfillment, where retailers could easily make efficient decisions about which carriers, distribution centers, and packaging types to use, even without perfect information about what those associated costs may be. In today’s world of constantly changing fuel, carrier, and materials costs, this type of technology can be revolutionary for a retailer’s reverse operation.
AI AND SUSTAINABILITY
Not only can AI be used in decision-making and cost-saving tools, it has the power to significantly reduce retailers’ environmental impact. At Optoro, we carefully track a metric we call Reuse Rate, which measures the percentage of our clients’ returned inventory that gets restocked, resold, or donated. This helps us understand how effectively our software – and the AI that powers it – transforms returns into forward opportunities, and directly correlates with increases in top-line revenue and decreases in returns costs for our clients.
One of the most impactful tactics retailers can take to further their sustainability goals starts with the most foundational level of AI – tracking data. Putting aside more advanced machine learning technologies, many retailers today lack basic visibility into how their operations impact the environment and how those impacts compare to similar companies in the field. Simple dashboards that track a handful of sustainability metrics can go a long way in helping executives understand how company actions affect environment outcomes. This is the first step retailers must take on their paths towards environmental excellence.
As AI constantly evolves and develops, the future of retail and returns is following suit. With the holiday season quickly approaching, the smartest retailers will invest in end-to-end reverse logistics software that harnesses AI to reduce friction, provide insightful data, and improve their returns for customers, supply chains, and the environment.
Optoro is the leading provider of returns technology for retailers and brands, using data science and real-time decision making automation to make returns better for customers, retailers, and the planet. From an easy online customer returns portal, to warehouse processing and resale software, we offer powerful solutions to improve outcomes across all points in the returns process. Learn more about Optoro’s solutions at www.optoro.com.
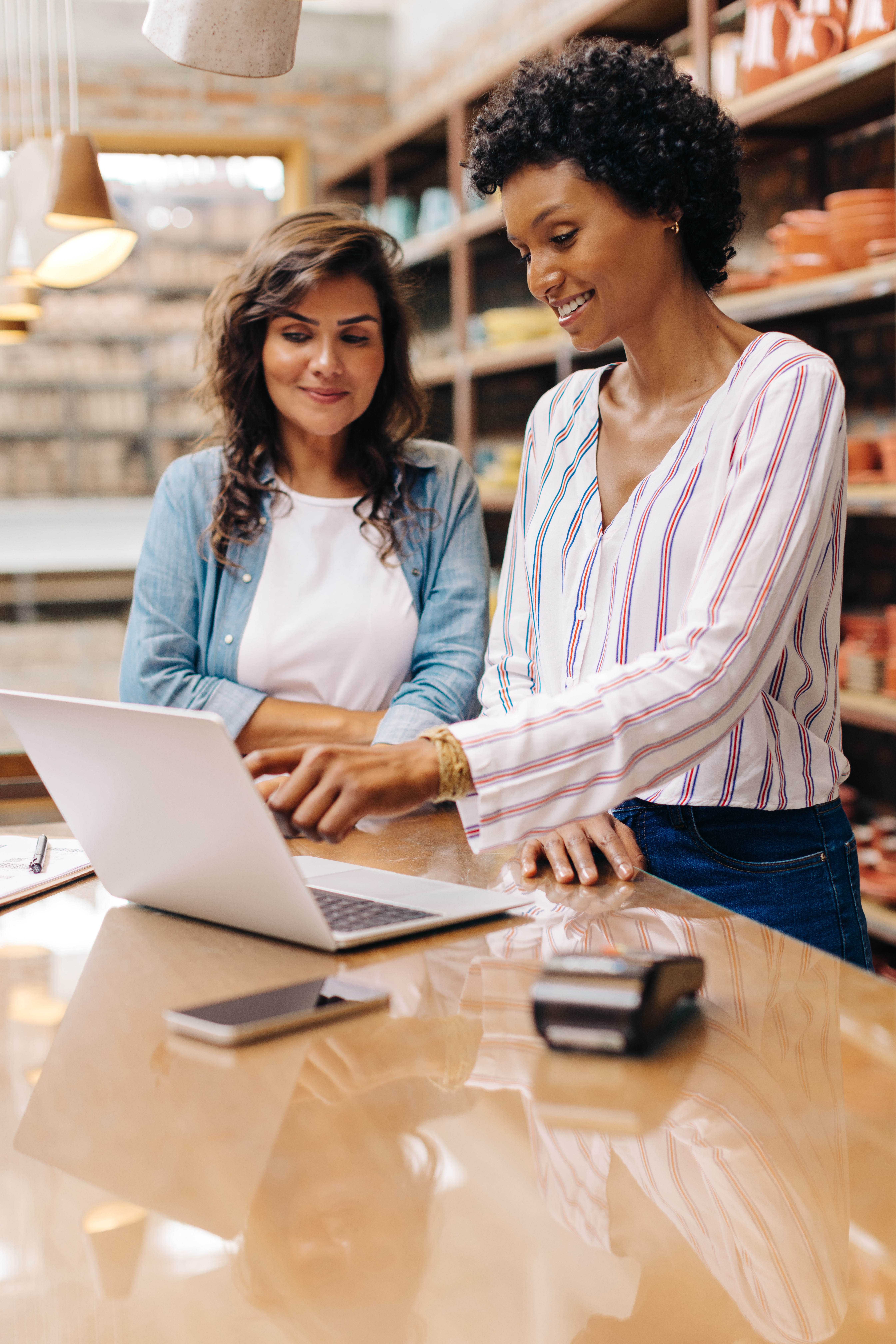
SOURCES
1. Calculated by Optoro Analytics, 2022
2. Calculated by Optoro’s Express Returns Carbon Impact Model, 2022
3. Calculated using Optoro’s Recommerce channels, 2022
Mike GanzMike is a leader in the data space, currently serving as Senior Director of Data & Analytics at Optoro. There he focuses his time on differentiating Optoro's products using advanced analytics and data science, ensuring that the most difficult returns problems retailers and the environment face are met with robust data solutions.
Prior to joining Optoro, Mike led analytics and data science engagements at Applied Predictive Technologies, later acquired by MasterCard. In that role he helped Fortune 500 companies in the retail and financial services space design, implement, measure, and forecast out the impact of business experiments.
Mike holds a B.S.E in Systems Science & Engineering from the University of Pennsylvania.