Is your Reverse logistic Process AI-Ready?
By Michael Kerstein , OnProcess Technology
Just a few years ago, artificial intelligence enjoyed limited popularity, mainly amongst scientists and tech enthusiasts. Still, lately, it feels like we’re watching the fourth industrial revolution unfold before our eyes at 5x speed, whereas AI has gone mainstream with the recent advancements in generative technology and the increased availability of interactive chatbots, allowing everyone with an internet connection to experience AI firsthand.
According to the 2023 MHI Annual Industry Report, artificial intelligence is now viewed as the most impactful innovation in the supply chain industry, jumping four positions compared to the year before.
Setting aside the media buzz, there is a good reason companies are putting AI at the forefront of future investments: the promise of revolutionizing how logistics work by reducing time spent on repetitive tasks, automating customer interactions, and analyzing huge chunks of data to provide valuable insight.
The successful implementation of AI will ultimately impact how supply chains are managed, delivering streamlined processes, improved customer satisfaction, and huge savings along the way, and despite the excitement generated by this emerging technology, the reverse logistics sector is yet to catch on.
According to a recent study OnProcess conducted in partnership with WBR Insights, only 35% of service supply chain companies currently have AI-powered asset recovery processes. And realistically, this will not change overnight.
The truth is that while everyone wants to hop on the latest technological breakthroughs, it is easier said than done.
Price, for instance, poses a significant barrier to entry and varies greatly on the business scope. Implementing an out-of-the-box solution to automate a single process could cost several thousand dollars while creating a custom system to manage your entire operations can go for millions. There are also additional ongoing expenses associated with hardware, maintenance, and updates to be considered.
Regardless of the initial investment, a recent IBM study also showed that few AI projects deliver the expected returns, with an average ROI of just 5.9%, lower than the 10% returns typically expected.
If this is the case, is it worth it to make the investment? The answer is Yes, but only if you do it properly.
Unsurprisingly, according to the same study, one of the key differentiators for success was the focus on data, with best-in-class companies achieving a 13% ROI.
The value of data in business is widely acknowledged, as artificial intelligence systems are trained on the data you feed them, it is crucial for the input to be of the highest quality.
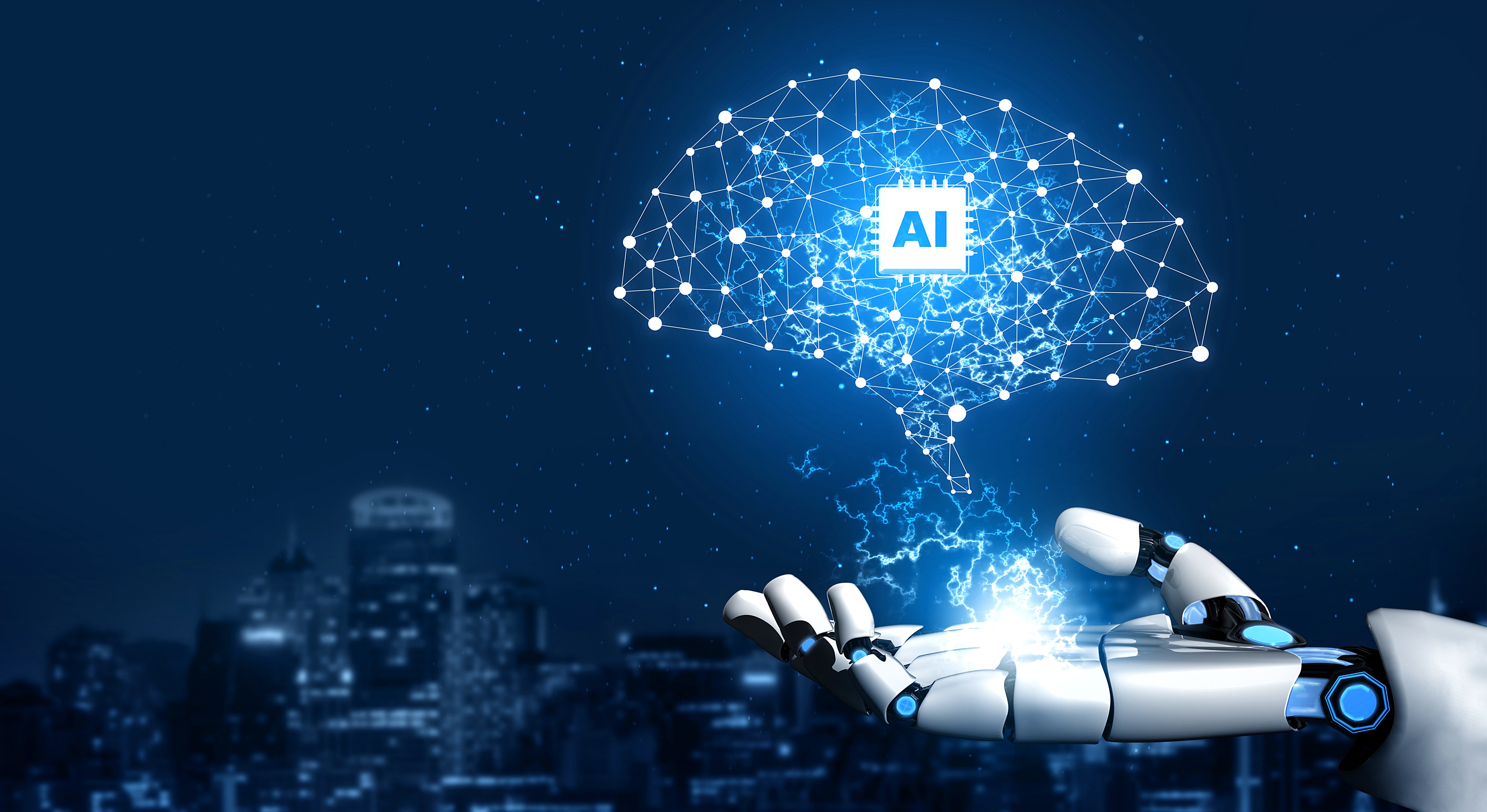
The stages for a successful implementation of AI in your returns processes are set on good data habits and on a solid digital foundation:
- De-Silo data. Internal organizations, software tools, transportation and warehouse partners, repair teams, scrap vendors, and customers all have their own metrics and technologies. Make it easy and appealing for the various stakeholders to actively share their data into a centralized pool of information to create a foundation for a predictive AI-powered returns process.
- Rationalize data. Evaluate your data and figure out which one is relevant for the output you want to achieve. Consider cleaning and normalizing your data before feeding it to the AI system to avoid time and resources spent on processing irrelevant data, thus ensuring it will be up and running as fast as possible.
- Ensure data is accurate. One of the biggest concerns in the AI world is accuracy - the degree to which an AI system produces correct outputs or predictions based on the given inputs or data. Training your model on inaccurate data will lead to inconsistencies and wrong predictions, and basing business decisions on faulty data can be time-consuming and expensive to fix.
- Feed real-time data to the algorithm. This allows for an increased speed of processing, with systems tracking trends and patterns as they are happening and flagging outliers. Real-time information unleashes the full potential of AI, allowing instantaneous alerts for issues and inefficiencies in the returns processes, empowering faster decision-making and cost savings along the way.
AI adoption is not as straightforward as some experts claim. However, the benefit for your reverse logistics processes is undeniable.
Let’s assume a company implements AI to improve the operation performance of the return process of old parts. If the system is properly implemented, it can provide the operational teams with reliable and real-time information on how the part needs to be dealt with, whether it needs to be refurbished or reused, decommissioned, or recycled. Resulting in fewer to no human errors, optimized time, and routes, leading to lower costs and improved inventory management.
With over 70% of companies forecasting to adopt AI into their supply chain processes in the upcoming 5 years, MHI reported; 2023 is the year of AI, and the decisions surrounding adoption are no longer an ‘if’ but a ‘when’.
After all, reverse logistics is trickier than one might expect. It’s not enough to have static plans for retrieving your parts. Things change fast, and a number of variables might alter in a second. That’s where AI - and the ability to enable intelligent, real-time decisions - comes in.
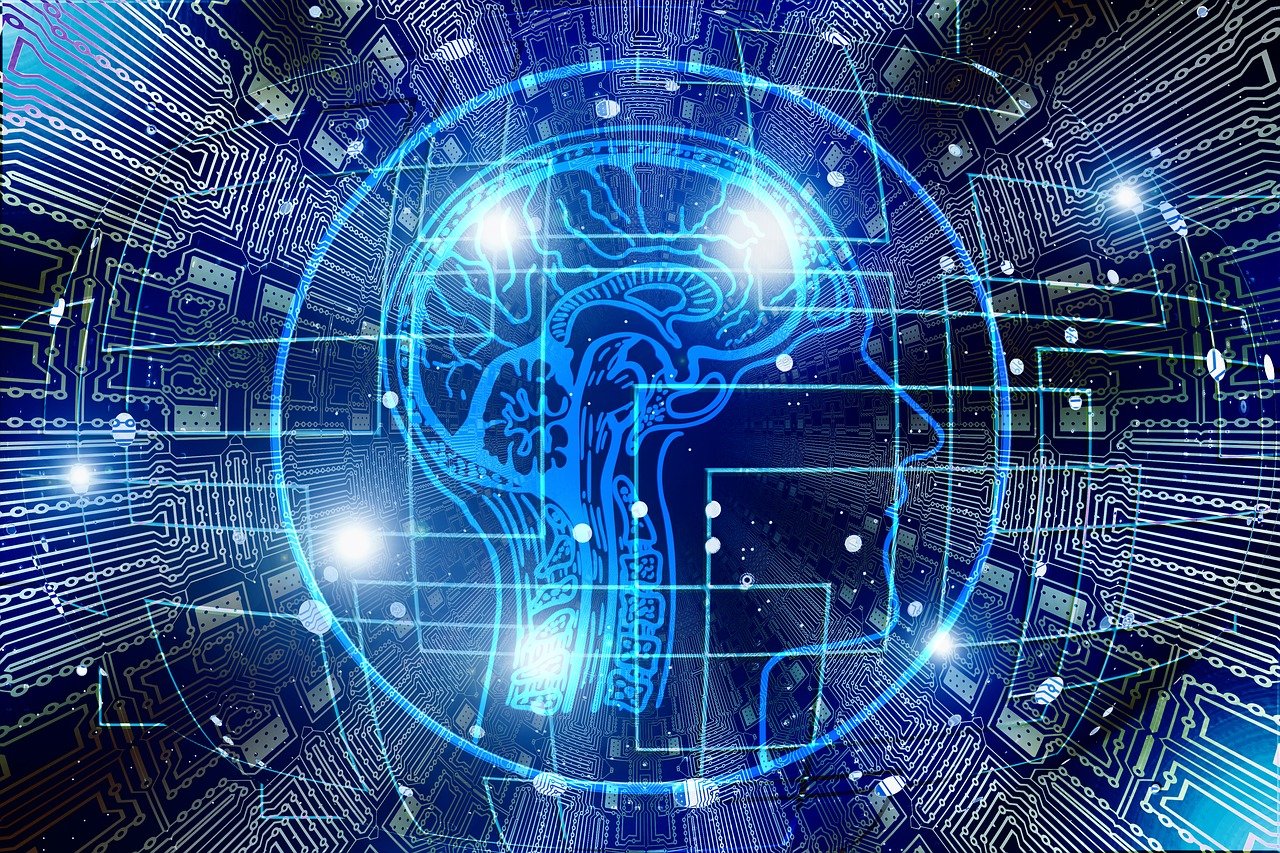
Michael Kerstein Michael Kerstein joined OnProcess Technology in May 2022 as a Chief Product Officer. He is responsible for the strategy, definition, and delivery of the OnProcess product portfolio. Mike has years of experience as a product leader with a passion for building products for high-growth technology companies that drive insight and value in data-rich industries. He has led the development, launch, and support of multiple successful Enterprise SaaS products in the Field Service, Mining, and Defense industries. Most recently, Mike led product at XOi Technologies, where he drove the product strategy and the launch of several new AI-driven data products.