From Data to Decisions: How AI Provides Valuable Reverse Logistics Insights to Drive Hyper-Efficiency
By Amit Gautam, Innover Digital
The global retail landscape is inundated with rising returns pressure, forcing organizations to reshape their supply chain models to accommodate space and strategy that can ensure a circular economy. Even though Reverse Logistics has been often neglected, an ever-expanding online retail market coupled with rising customer expectations are continually straining existing supply chains to focus on reverse logistics.
As an example, a recent report cited that almost one third of total online holiday sales ($234.9 billion) in 2020 across the US are expected to be returned. Therefore, in the current context, organizations looking to stay competitive and profitable need to innovate and adapt to this rapid shift.
From an organizational perspective, even though reverse logistics practices can cut down costs and increase customer satisfaction, its implementation can pose several challenges in terms of re-strategizing and realignment of supply chain and operations.
Challenges in Retail Logistics Implementation
In essence, Reverse Logistics entails returning the original products back to manufacturers. However, to circumvent the complexities involved in managing these returns many retailers and brands often choose to outsource the entire reverse logistics process.
On the other hand, firms that decide to build their own reverse logistics need to have robust capabilities in place to efficiently manage the extra supply chain load. Given the complex interrelationships between stakeholders in the supply chain, it puts enormous pressure on the network. In this scenario, closing the loop can often mean more costs involved in inventory, freight, and labor. On top of this, factors like product depreciation and recovery losses also further add to the liabilities.
It becomes clear that optimizing these processes requires supply chain visibility that can be only be achieved with proper real-time information flow. The lack of verified data can lead to organizations finding data discrepancies and lack of trust about confidentiality. This subsequently hinders both reverse logistics implementation as well as accurate measurement of circular economy models.
In addition to the information flow, efficient reverse logistics also necessitate a deep analytical view of the supply chain activities. A panel of analytical experts and process SMEs with deep domain knowledge in the organizational returns management are required to understand the supply chain business issues and customer behaviors.
The team must be supplemented with an insights and decision support platform that can integrate and monitor transactional data from external and internal sources. The platform must be able to separate issues automatically from the mass and generate insights and subsequent actions needed to improve the end-to-end process. Gaining these valuable insights can make the entire process substantially more effective and economical.
Recent developments indicate that with the post pandemic increase in momentum of Industrie 4.0, organizations are waking up to the power of intelligent assets and the role they play to enhance their circular business efforts.
AI-powered Tools Enabling Reverse Logistics
Analyzing supply chain data in the manufacturing sector has been helpful in reducing waste and optimizing energy consumption. There are examples where using AI-enabled digital technologies have helped papermaking facilities unlock tangible business values including reduction in the usage of raw materials, increase in process efficiency by about 2.5%, and lowering of energy input by 35%.
Organizations involved in waste recycling and recovery are also using different technologies powered by AI to optimize their operations. The following instances highlight the progress of AI in the world of reverse logistics:
- The waste management robot, Clarke, uses advanced vision system and deep learning to reduce waste picking cost by 50%.
- Waste treatment plant has leveraged AI-enabled image recognition and machine data analysis leading to an increase in recycling share by 10%.
- A smart waste factory in Austria has interconnected waste data with machine controls and a sensors integrated digital platform to increase waste treatment efficiency and obtain greater rates of recycling and recovery.
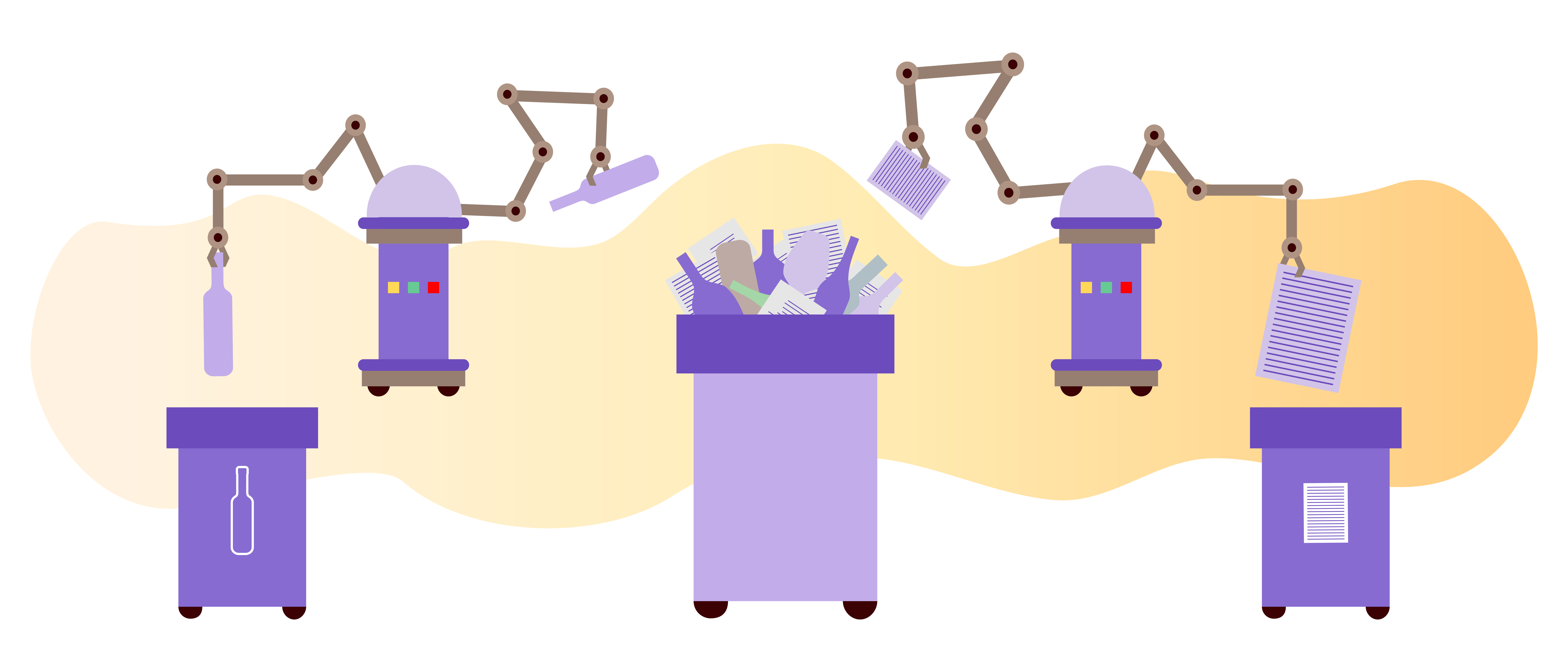
Elsewhere AI/ML powered retail platforms are leveraging machine learning with custom business logic to understand demand and product routing. The platform uses algorithms to figure out if items can be re-shelved or sent off to an off-price alternative retailer. The system is capable of analyzing the daily sales figures for each item in tandem with the actual market demands to foster circular logistic decision making.
With the vision to maintain circularity, a combination of different complementing technologies can help manage a product’s lifecycle even if it has to go through reverse logistics. There are several examples to consider in this direction:
- AI-enabled 3D-printing can better control inventory and product efficiency.
- Waste analytics ensure the materials’ value is sustained across the economic circle.
- Tracking technologies – such as RFID, NFC sensors, and GPS improve product traceability in real-time.
- Predictive analytics combines the data from across the supply chain to prepare the manufacturers about the returns. Additionally, defects analysis helps them make effective decisions related to regeneration, restoration and/or re-manufacturing of the product.
AI-enabled Hyper-efficiency for Future-Forward OrganizationsIndustries in general are still under-utilizing products, filling garbage lands, and failing to recycle products. However, this is not in sync with current trends and practices where efficiency and concepts of lean manufacturing are fast becoming the keys toward future sustainability. Future facing organizations would do well to be aware of the benefits of being sustainable as with growing consumer consciousness, sustainable businesses are increasingly being looked at favorably and have higher chances of retaining customers and building a positive brand image. Business leaders across the spectrum must realize how to create value from reverse logistics to enable a circular economy.
As with every aspect of business, technology and innovation can make a big differentiating factor here. To this, different AI-enabled technologies have already opened up opportunities for organizations to not only create value from returned products but also accelerate operational efficiency in the process. And with hyper-converged infrastructure, software-defined networks, virtualized transactions, cloud-enabled information sharing, and transparent business models of the future, building a hyper-efficient and cost-competitive organization will no more remain a concept. On the contrary, it will be an achievable mission for brands looking to thrive in a new era by supporting efforts of regeneration, building resilience and making the business fit for the long term.
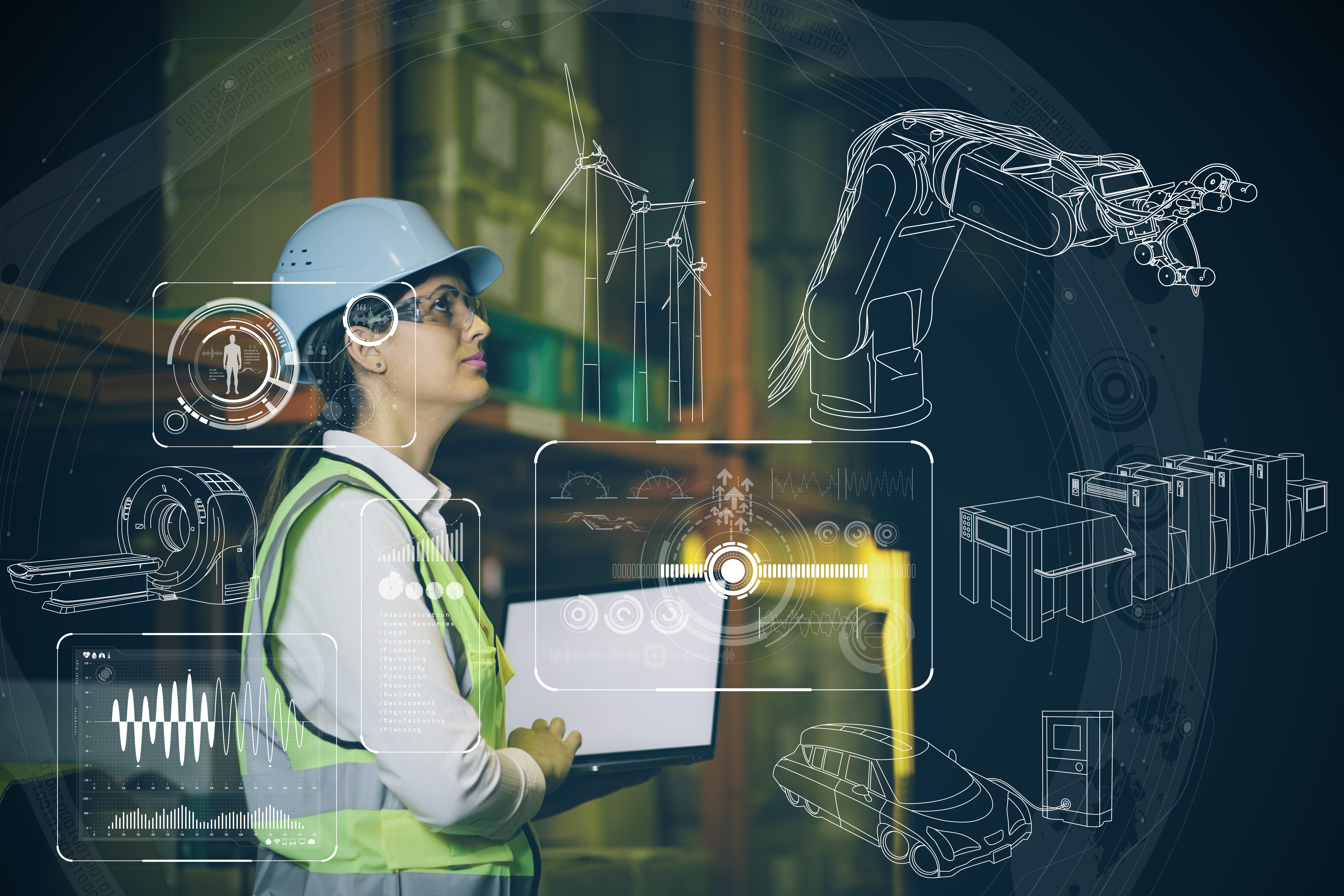
Amit GautamIn his role as President and Chief Strategy Officer for Innover, Amit Gautam has responsibility for all aspects of the company’s product & services strategy & execution, as well as its financial performance and growth.
Amit has a relentless focus on Growth and Innovation and holds a strong personal commitment towards “Outcome-Driven” Digital Transformation for businesses. Amit collaborates with the C-suite executives of Fortune 1000 companies and guides them to adopt a digital-first mindset, delivering bold transformations and exceptional experiences.
Prior to Innover, Amit worked with firms like GE and Cognizant in various leadership roles. Amit studied Data Science at Harvard and holds a Bachelors Degree in Engineering from India.